Machine Learning Density Functional Theory
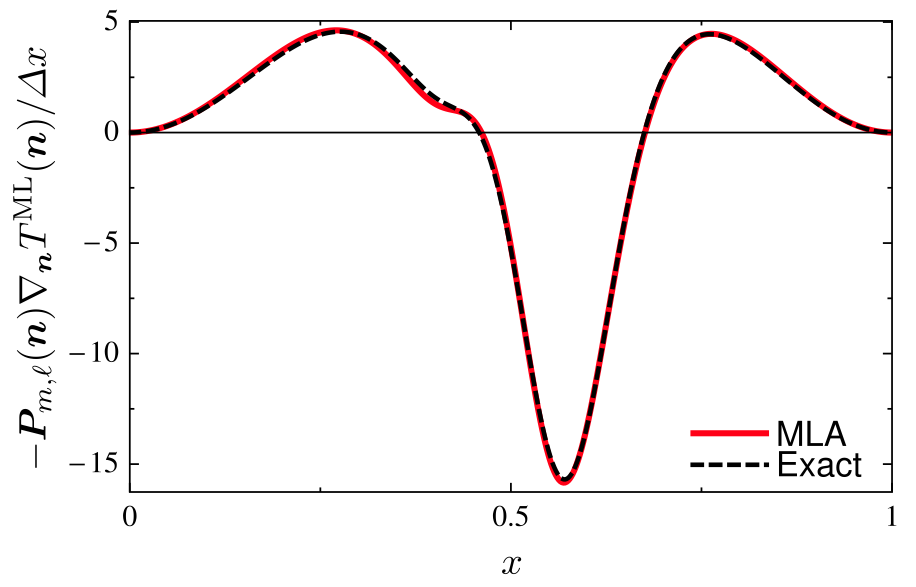
Our group was the first to apply robust machine learning (ML) methods to directly 'learn' density functionals. ML is a powerful method capable of learning functions of high-dimensional spaces via induction.
Most DFT research solving the kinetic energy from Kohn-Sham equation. However, it is not needed in principle from the theorems of DFT. If the kinetic energy of such electrons were known as a functional of density, then a single equation could be solved for the exact self-consistent density and the energy extracted. There would be no need to solve the KS equations, and the computational bottleneck would presumably become solving the Poisson equation. Thus DFT calculations would become much faster than they already are.
In a proof-of-principle [Phys. Rev. Lett. 108, 253002 (2012)], we used ML to approximate the kinetic energy functional of particles confined to a one-dimensional box with chemical accuracy. We are actively researching extending this method to 3d systems. Applying this method to real complex systems would enable highly accurate orbital-free density functional calculations and ab-initio molecular dynamics simulations.
Publications
Funding
We graciously acknowledge support from the NSF Grant No. CHE-1240252.